3D Shape Generation and Completion through Point-Voxel Diffusion
Linqi Zhou1 Yilun Du2 Jiajun Wu1
1 Stanford University; 2 MIT;
ICCV 2021
Paper | Code |
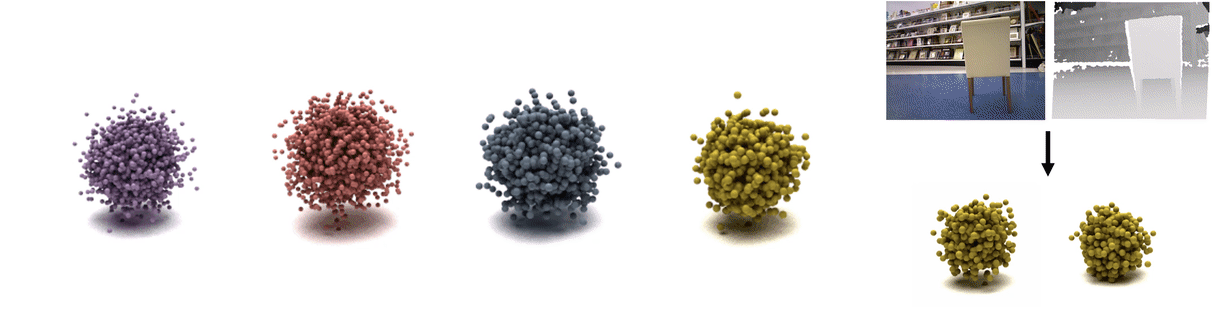
Overview
We propose a novel approach for probabilistic generative modeling of 3D shapes. Unlike most existing models that learn to deterministically translate a latent vector to a shape, our model, Point-Voxel Diffusion (PVD), is a unified, probabilistic formulation for unconditional shape generation and conditional, multi-modal shape completion. PVD combines denoising diffusion models with the hybrid, point-voxel representation of 3D shapes. It can be viewed as a series of denoising steps, reversing the diffusion process from observed point cloud data to Gaussian noise, and is trained by optimizing a variational lower bound to the (conditional) likelihood function. Experiments demonstrate that PVD is capable of synthesizing high-fidelity shapes, completing partial point clouds, and generating multiple completion results from single-view depth scans of real objects.
Generation and Completion
Our model can perform fully unconditional generation and conditional generation, a.k.a. shape completion. For the following figure: (left) our generation result, (right) depth maps, sampled partial shapes, and our completion.
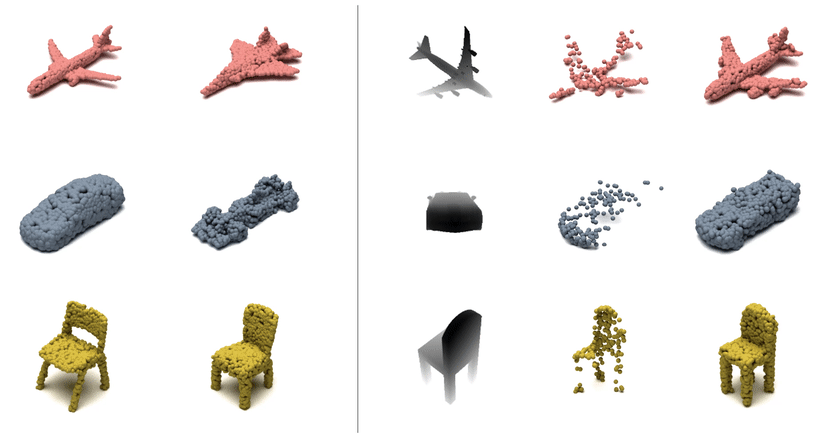
Generation | Completion |
Multimodal Completion
On ShapeNet, our model gives considerably diverse completion. For the following figure: (top) completion from input view, (bottom) our completion. The depth image of the bottom of a chair is shown on the left.
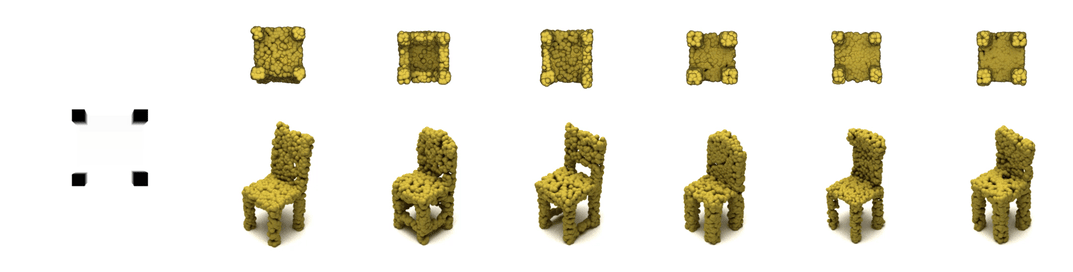
Input Depth | Possible Completion |
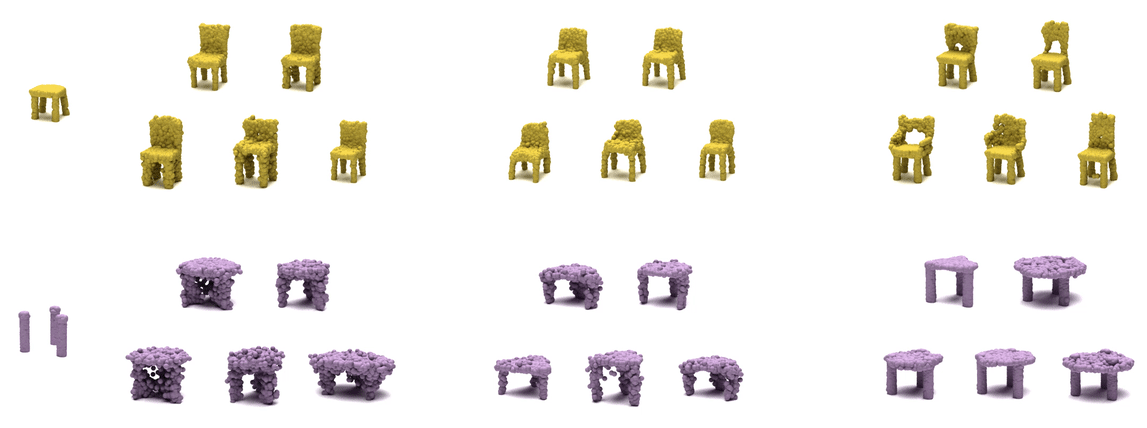
cGAN | KNN-latent | PVD (Ours) |
Our pretrained model on ShapeNet can also perform well on real dataset, Redwood 3DScan. Here, the left two examples show more complete views of a chair and a table, whose completion are stable. The right two examples show more uncertain views of a chair and a table, and their completion show variability. Within each example, (top) 3 completion from input view, (bottom) 3 full completion.
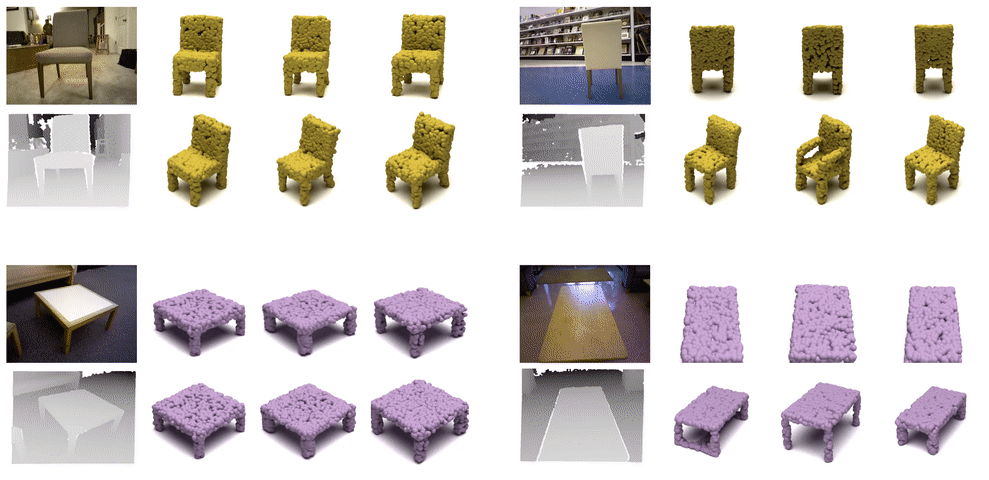
RGB-D | Possible Completion | RGB-D | Possible Completion |
Citation
@inproceedings{Zhou_2021_ICCV, author = {Zhou, Linqi and Du, Yilun and Wu, Jiajun}, title = {3D Shape Generation and Completion Through Point-Voxel Diffusion}, booktitle = {Proceedings of the IEEE/CVF International Conference on Computer Vision (ICCV)}, month = {October}, year = {2021}, pages = {5826-5835} }
Please send any questions to Linqi Zhou and Yilun Du.